Research
Overview
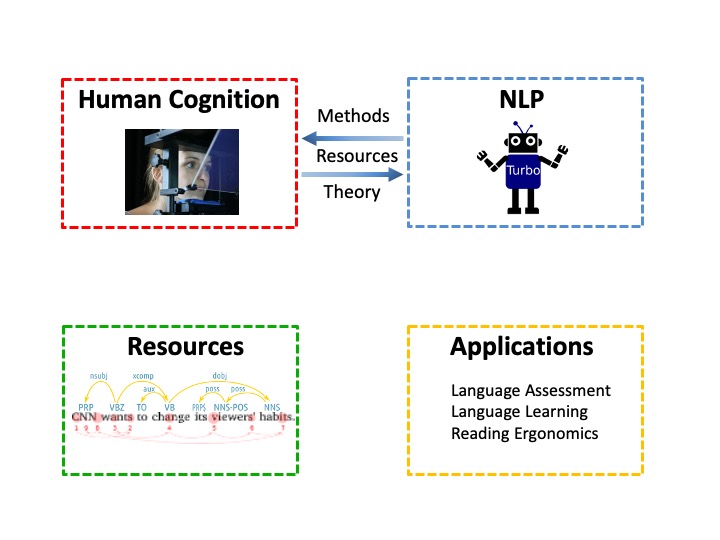
Our research aims to create a new conceptual and computational framework for integrating the scientific study of human language processing with the field of Natural Language Processing (NLP). It puts forward the idea that NLP systems and machine learning techniques can be highly instrumental for modeling how humans learn and process language. In a complementary vein, it posits that insights from human language learning and behavioral and neural data from human language processing will be key to the development of NLP systems which can process language effectively.
To realize this framework, we go beyond traditional methodologies in linguistics and psychology, and study the human language ability using novel experimental methods which leverage the versatility of NLP and machine learning techniques. At the same time, we develop computational models for existing and new NLP tasks by drawing on human cognition and behavior. Consequently, our research has four underlying goals.
- Advancing our scientific understanding of the cognitive foundations of human language processing via computational modeling and experimental studies.
- Improving NLP by developing NLP systems which process text in a human like fashion.
- Creation of high quality resources which broadly support research at the intersection of NLP and Cognitive Science.
- Development of real world applications for language assessment, language learning and reading ergonomics.
In the long term, our research aims to contribute to fundamental shifts in the scope and nature of NLP research as well as scientific research of human language processing. In particular, it aims to contribute to extending NLP beyond its traditional tasks, and help position it as a core component in the scientific inquiry of language. This transition increase the cross fertilization between NLP and other fields concerned with language science, including Linguistics, Psychology and Education. The utilization of large scale behavioral and neural data and cognitively driven modelling will be instrumental for the future advancement of NLP, which will crucially rely on the ability to reproduce deep, human like language processing and understanding in machines. Progress on these fronts will further feed into a wide range of new cognitive language applications with a high potential for societal impact.
Human Language Processing
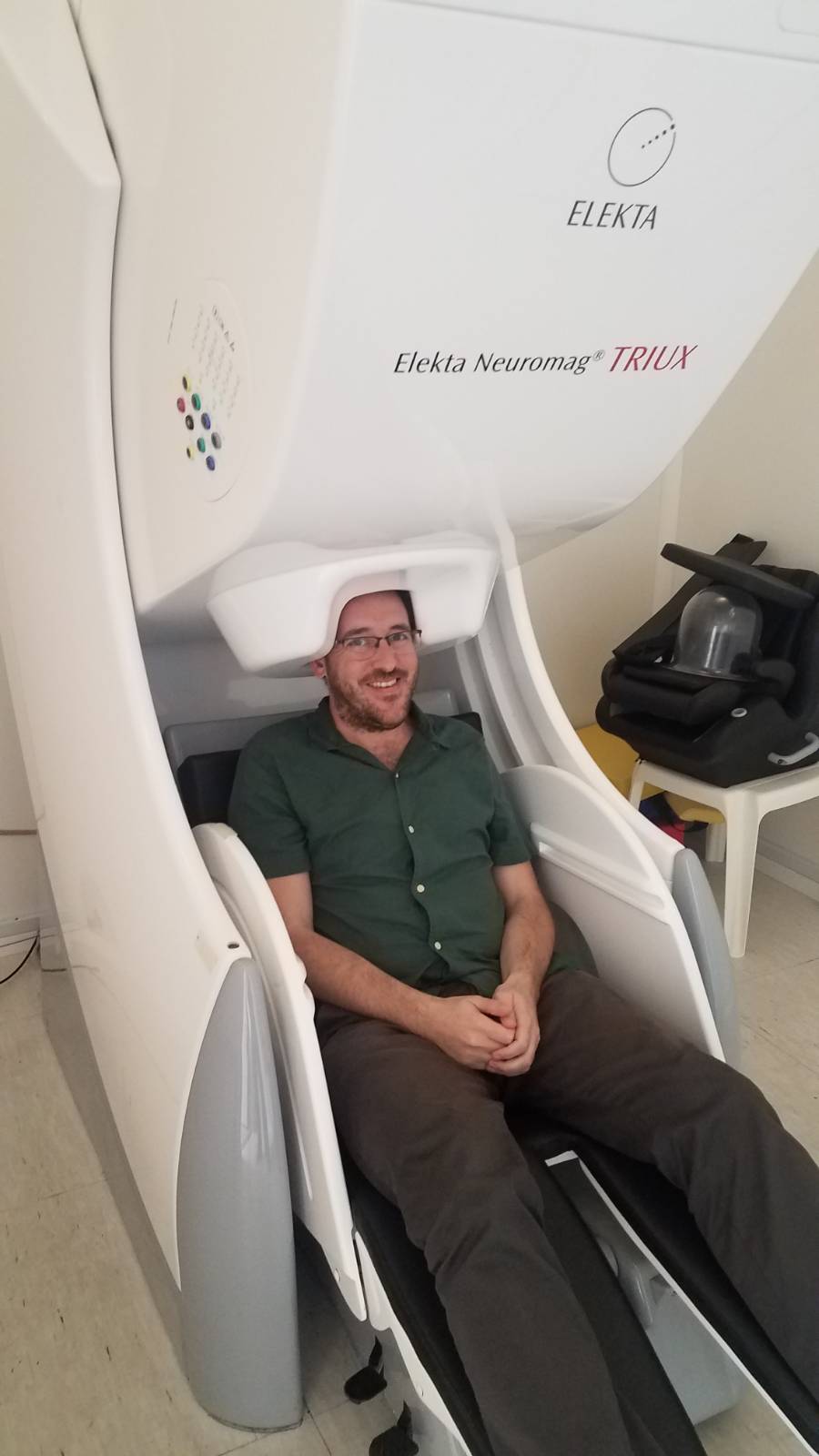
As you are reading this page, your eyes are moving over the text in a way that is highly informative of your linguistic knowledge, goals and cognitive state. A major research direction we are pursuing uses behavioral traces of eye movements during reading for developing a theory and computational models of the cognitive mechanisms that govern human language learning and processing. In the framework of this research, we design and carry out eye-tracking experiments which enable studying what eye movement patterns during reading can reveal about the reader and how they interact with text. In a closely related line of research we study neural signals obtained with Magnetoencephalography (MEG) and Electroencephalography (EEG) during language comprehension.
Cognitively Driven Natural Language Processing
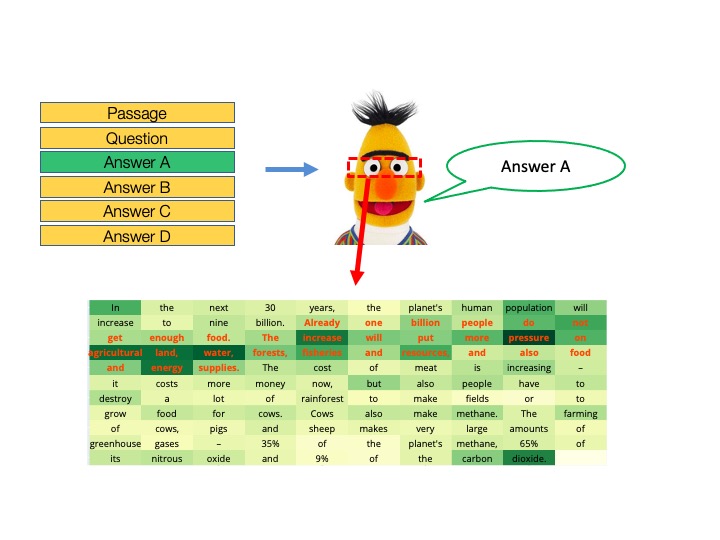
In a complementary thread to our investigation of human language processing, we design NLP models that absorb and process text in a human like fashion. In this context, we explore leveraging human behavioral and neural data as a source of information which can guide the behavior and improve the performance of NLP models. In addition to integrating human behavioral and neural data in NLP tasks, we are also working on NLP models that are designed to learn language in a way similar to humans. Humans are able to learn language without explicit annotations traditionally used in NLP, among others, by taking advantage of perceptual context. We develop NLP models that learn language similarly, by utilizing the visual context of utterances to facilitate language interpretation and learning. We are also interested in multilingual NLP and its relation to the cognitive representations that support multilingualism in humans.
Resources and Methodology
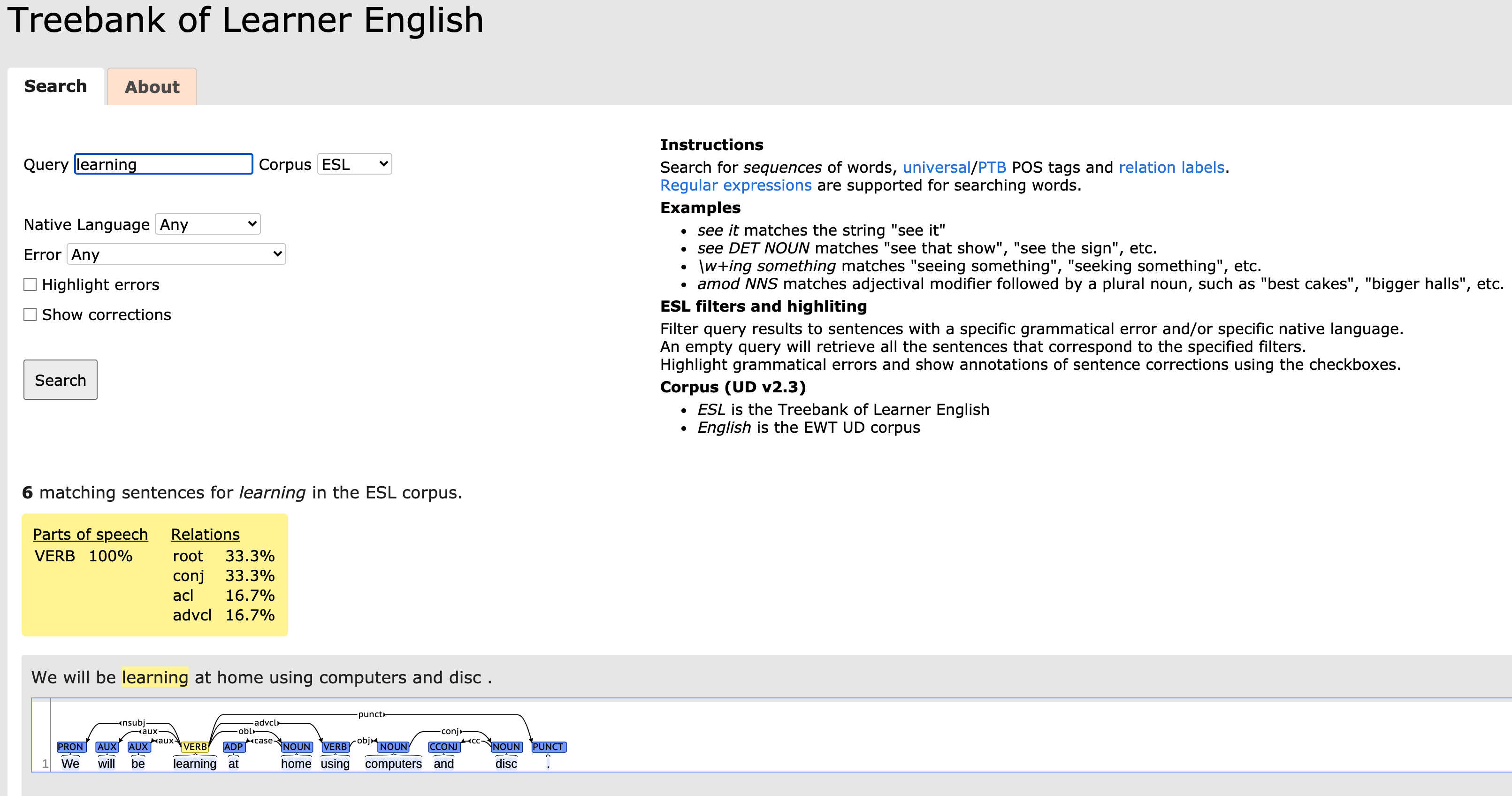
We believe that advances in NLP and Cognitive Science are driven not only by new models and theories but also, and perhaps even more so by high quality resources. To enable our research, we are creating datasets which facilitate addressing new scientific questions and NLP tasks, many of which are difficult to study using existing resources. In addition to creating new resources we are also interested in the methodology of dataset development, and are studying cognitive biases which affect human annotations and inter-annotator agreement.
Language Applications
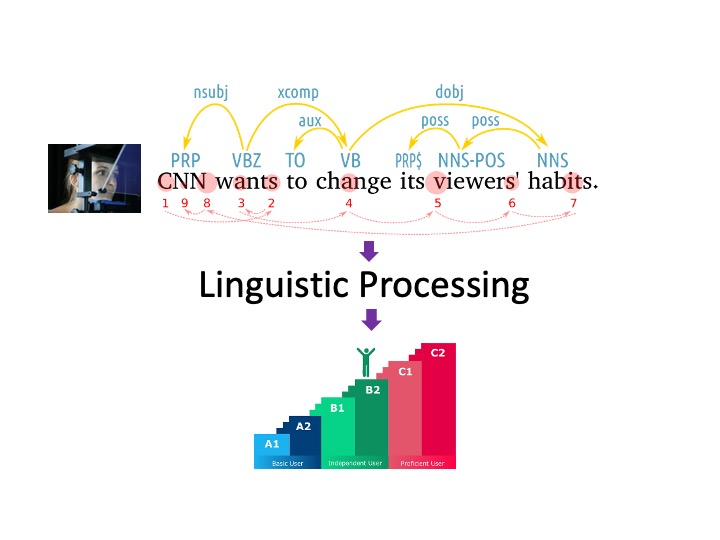
We aim to leverage our research for deploying real world applications for language assessment, language learning and reading ergonomics. For example, departing from traditional language proficiency evaluation methods, we have developed EyeScore, a measure of linguistic proficiency for ESL readers which is derived as a byproduct of ordinary reading. Extending this application, our ongoing research aims to enable detailed evaluations of linguistic knowledge based on eye movements in reading, determining text readability, as well as adjusting text difficulty to the linguistic proficiency and text understanding of the reader in real time.